Histology 2.0 – Precision histology of pathological processes using artificial intelligence
Prostate Cancer
Of all cancer types, prostate cancer has the highest incidence, meaning that there are more new cases of prostate cancer every year than any other specific type of cancer [1]. While it is not one of the more dangerous cancer types, its high incidence still results in a high mortality (deaths per year and population) with only lung and intestine leading to more deaths and pancreas (the most deadly cancer type) being about equal [1].
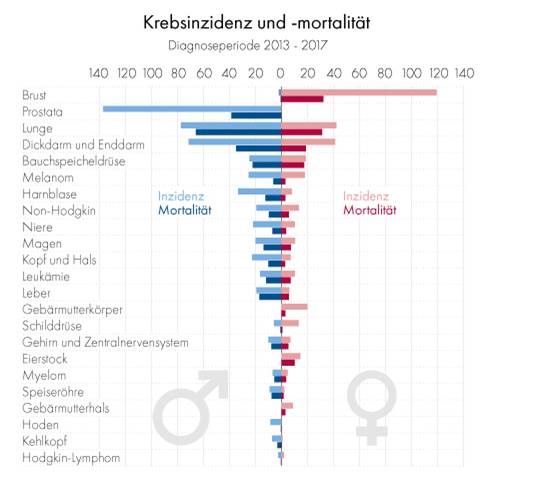
Prostate cancer is a particularly complex issue as it is well treatable when discovered early and there are multiple approaches from ‘watchful waiting’ to complete prostate removal (prostatectomy) [2]. However, as soon as this cancer metastasizes to other sites of the organisms, it is hard to eliminate. A particular difficulty stems from the diversity of various forms of prostate cancer, with some being very aggressive and fast growing, while others proceed only very slowly so that surgical removal would not be necessary. However, in most cases it is not known whether a detected prostate cancer is aggressive or rather moderate. Therefore, most patients and physicians, at least in Austria, decide for surgical removal of the prostate, which is a highly unpleasant treatment with severe side effects such as erectile dysfunction and temporary incontinence. It is assumed that up to 40% of prostate cancer patients would not need the harsh treatment of surgical removal of the prostate. However, unfortunately, it is difficult to identify, which patients belong to this 40%. Simply spoken, we could state that there is not one prostate cancer but maybe 20 different ones, with many more subtypes – depending on the specific combination of genetic, molecular, or cellular aberrations. Therefore, proper diagnosis and identification of the specific form of cancer is indispensable in making the right decision for each individual patient.
Prostate cancer is a particularly complex issue as it is well treatable when discovered early and there are multiple approaches from ‘watchful waiting’ to complete prostate removal (prostatectomy) [2]. However, as soon as this cancer metastasizes to other sites of the organisms, it is hard to eliminate. A particular difficulty stems from the diversity of various forms of prostate cancer, with some being very aggressive and fast growing, while others proceed only very slowly so that surgical removal would not be necessary. However, in most cases it is not known whether a detected prostate cancer is aggressive or rather moderate. Therefore, most patients and physicians, at least in Austria, decide for surgical removal of the prostate, which is a highly unpleasant treatment with severe side effects such as erectile dysfunction and temporary incontinence. It is assumed that up to 40% of prostate cancer patients would not need the harsh treatment of surgical removal of the prostate. However, unfortunately, it is difficult to identify, which patients belong to this 40%. Simply spoken, we could state that there is not one prostate cancer but maybe 20 different ones, with many more subtypes – depending on the specific combination of genetic, molecular, or cellular aberrations. Therefore, proper diagnosis and identification of the specific form of cancer is indispensable in making the right decision for each individual patient.
The diagnosis of prostate cancer
There are certain chemical biomarkers that are measured in the blood and which are a good indication of the state of the prostate, the most prominent being PSA (prostate-specific-antigen) which slowly increases with age – showing a rapid rise in prostate cancer [5]. However, most of these values can vary highly even between healthy individuals, leading to false negative or positive diagnosis.
Therefore, an often-employed technique is a needle biopsy, in which a wide needle is inserted into the prostate to obtain a tissue sample like a drill core sample [6]. This can be viewed under the microscope to identify possible cancer foci. However, this is also where the problems begin, because if the needle does not hit a cancer focus, then the pathologist might not be able to find the right diagnosis. Taking multiple needle biopsies increases the likelihood of finding a present cancer, but also leads to more pain for the patient and more damage to the prostate, which can also act as an escape route for metastasizing prostate cancer cells.
Better diagnostics
This is where our project sets in. Our group has for a long time investigated the links that exist between cancer and the process of inflammation. These are often strongly associated as many cancers lead to a chronic inflammatory condition and vice versa inflammatory processes lead to a higher risk of developing cancer [7]. The molecular and cellular changes, which are caused by inflammation or malignant transformation of cells, are often not visible to the human eye, but can be measured either by looking for tiny alterations in the tissue architecture, or by looking for the presence of specific signaling molecules and their interactions with each other.
Artificial intelligence to support diagnostics
The human ability of pattern recognition is outstanding, in particular for things, which were important throughout evolution such as recognizing faces or animals. However, a precise evaluation of biological samples in the microscope and recognizing disease states simply by the tissue architecture, is much more difficult and requires many years of training and experience as pathologist. Nevertheless, even for trained specialists, visual evaluation of a specimen is a rather qualitative process than a quantitative assessment. This is the field, where computer-based algorithms and detection routines can outperform humans, as they can measure image data in a precise and quantitative manner, providing an unbiased and constant evaluation of tissue sections. This concept can be pushed even further by applying artificial intelligence. In this case, machine learning strategies are used by exploiting training sets of images obtained from healthy and diseased tissue. Thereby, the computational system can develop an automated classification and evaluation scheme, identifying features that cannot even be seen by a human, but which are hidden within the tiny but re-occurring changes in the image data. Hence, if the dataset is good, even the tiniest differences can be identified and evaluated by a good algorithm. Certainly, these methods cannot replace the expertise of trained pathologists, but they can provide additional and quantitative data serving as highly valuable decision support.
In our project, we are collaborating with KML-Vision, a company located in the city of Graz, Austria, which offers artificial intelligence support to the scientific community, as well as pathology departments. They provide many different approaches which make it possible to automatically detect and evaluate various biological processes in images and even provide a routine to detect COVID-19 in lung X-rays (see further reading). Together, we investigate the possibilities that AI routines offer for the evaluation of prostate-cancer images.
Multi-fluorescent staining
The current routine in staining of tissue samples has not changed over decades. It includes two chemical dyes, Hematoxylin that stains for the cell nuclei, and Eosin which stains many proteins and therefore makes the tissue itself visible [8]. While this gives a good overview over tissue architecture, it does not provide any information on present biomarkers or other molecules. Hence, an antibody-based staining against a specific molecule is often added. However, normal bright field microscopy is limited to one, maybe two molecular targets and this is often not enough to get a full understanding of the occurring processes. To overcome this limitation, fluorescent antibody stainings are used, which are frequently employed in scientific research, and are also increasingly applied in clinical situations. Fluorescence is a property of some molecules in which you can illuminate them with light of a specific wavelength (color) and it will re-emit light of another specific wavelength (color). There are different fluorophores (fluorescent substances) spanning the entire spectrum of visible light (see further reading), and as their emitted light is always restricted to a certain spectrum of light, multiple of them can be used simultaneously to stain different targets within the same tissue, allowing the researcher to correlate them and find out how they interplay.
Classically, this allows staining for ~four different targets, because the use of more leads to problems as the spectra of these fluorophores overlap and detected signals can no longer be assigned to a specific target. However, there are ways around that problem. Knowing the spectrum of each fluorophore, spectral scanning and unmixing algorithms can be applied to isolate each individual color, and even detect background and tissue-autofluorescence, giving us a much wider range of simultaneous targets [9].
In this project, we are developing and employing exactly that, with the aim of simultaneously staining up to eight molecular targets within prostate cancer samples. This allows achieving a deeper analysis of crucial molecules and their interactions – and thus a better understanding of biological processes that drive prostate cancer development.
This project was made possible by the Austria based funding agency FFG under their BRIDGE – YOUNG SCIENTISTS project line. We are very grateful for their trust and support, especially in the troubling times of Covid-19.
Further reading
- https://www.cancer.org/cancer/prostate-cancer.html
- Site of the American cancer society on Prostate cancer, providing a vast amount of information on the disease, diagnosis and treatment.
- https://www.kmlvision.com/
Website of our collaboration partners KML Vision - https://www.fpbase.org/spectra/
One of many available spectra viewer in which the absorption and emission spectra of a large amount of available fluorophores can be displayed. - https://www.ffg.at/
The website of our funding agency, the FFG
Sources & References
- http://www.statistik.at/web_de/statistiken/menschen_und_gesellschaft/gesundheit/krebserkrankungen/index.html
- https://www.cancer.net/cancer-types/prostate-cancer/types-treatment
- Paller CJ and Antonarakis ES. Management of biochemically recurrent prostate cancer after local therapy: evolving standards of care and new directions. Clin Adv Hematol Oncol 2013; 11: 14–23.
- Schrijvers D. (2007) Androgen-Independent Prostate Cancer. In: Ramon J., Denis L.J. (eds) Prostate Cancer. Recent Results in Cancer Research, vol 175. Springer, Berlin, Heidelberg. https://doi.org/10.1007/978-3-540-40901-4_14
- Dejous C and Krishnan UM. Sensors for diagnosis of prostate cancer: Looking beyond the prostate specific antigen. Biosensors and Bioelectronics 2020, https://doi.org/10.1016/j.bios.2020.112790
- https://www.cancer.org/cancer/prostate-cancer/detection-diagnosis-staging/how-diagnosed.html
- Hoesel B, Schmid JA. The complexity of NF-κB signaling in inflammation and cancer. Mol Cancer. 2013;12:86. Published 2013 Aug 2. doi:10.1186/1476-4598-12-86
- https://en.wikipedia.org/wiki/H%26E_stain
- Grecco HE, Imtiaz S, Zamir E. Multiplexed imaging of intracellular protein networks. Cytometry A. 2016 Aug;89(8):761-75. doi: 10.1002/cyto.a.22876. Epub 2016 May 16. PMID: 27183498.